Computational pathology for nephropathology
DOI: https://doi.org/10.47184/tp.2023.01.02Digitisation of pathology enables computational pathology. Due to their excellent performance, deep learning-based systems are used primarily. In computational nephropathology, the focus of many studies is on large-scale extraction of comprehensible quantitative data from histological structures. The resulting data can be used for various downstream analyses, including prediction of the disease course. Such systems could significantly support nephropathological diagnostics in the future.
Keywords: Pathomics, Segmentation, Feature Extraction, Digital Pathology
Introduction
Nephropathology is a highly specialised area of clinical diagnostic pathology that focuses on biopsies of non-neoplastic kidney diseases. The diagnostic workup of a kidney biopsy typically involves integrating information from many tissue sections, stains and imaging modalities (light-, fluorescence- and electron microscopy) to assess a variety of defined lesions or injury patterns. These are often combined in semi-quantitative histological scores that are relevant to both formulate a final diagnosis and provide valuable, sometimes actionable information to clinicians. Important examples of such scores are the Banff-Classification of kidney allograft pathology [1] or the Oxford-classification of IgA-nephropathy [2]. However, the usefulness of these scores might sometimes be limited due to inter-observer variability and time required to perform the scoring.
Like the rest of pathology [3], nephropathology is undergoing digitisation [4] and studies on the use of artificial intelligence (AI)-based systems in nephropathology are published at an increasing rate. Most research uses deep learning, a subdomain of AI-research that makes use of deep artificial neural networks [5]. A particular focus in computational nephropathology lies on extraction of understandable quantitative data from histology that are used in a variety of downstream analyses. Typically, precise segmentation of histological structures (i. e., delineation of their borders), such as glomeruli or tubular cross sections is initially targeted. Segmentation then forms the basis for extracting structure related data, such as the area covered by, shape of, or the number of specific cells within the segmented structure. The next section highlights selected studies performing such analyses in kidney biopsies, focusing on recent advances.
Quantifying kidney histology using deep learning
The first multiclass segmentation model for nephropathology was trained to delineate several structures (glomeruli, tubuli, vessels and interstitium) with several classes (e. g., sclerotic and non-sclerotic glomeruli) in kidney allograft biopsies [6]. Data derived from segmented structures correlated well with pathologist derived Banff-lesion scores, illustrating the relevance of this approach. Studies performing segmentation of other structures, particularly peritubular capillaries [7] and kidneys from animal models [8] quickly followed, providing similar quantitative data extraction.
The downstream analysis of such datasets is particularly interesting when relevant clinical information is available. It has been shown that morphometric data derived from biopsies of IgA-nephropathy cases are independent predictors of renal long-term survival. A morphometric disease progression from healthy to end stage kidney disease can be inferred from the same data by performing a pseudotime trajectory analysis, a method developed for single cell transcriptomics data [9]. By going back to the images of the individual structure along the trajectory it is possible to assess its plausibility and identify specific morphological variations that might not directly be obvious from the structure related data (e. g., changes in the shape of the glomerular tuft might correspond to segmental sclerosis or cellular crescents). As the number of features extracted per histological structure increases, their analysis becomes more complicated and analysis techniques from the OMICS sciences become relevant for quantitative histopathology. This opens a novel research field within computational pathology: pathomics (Figure 1).
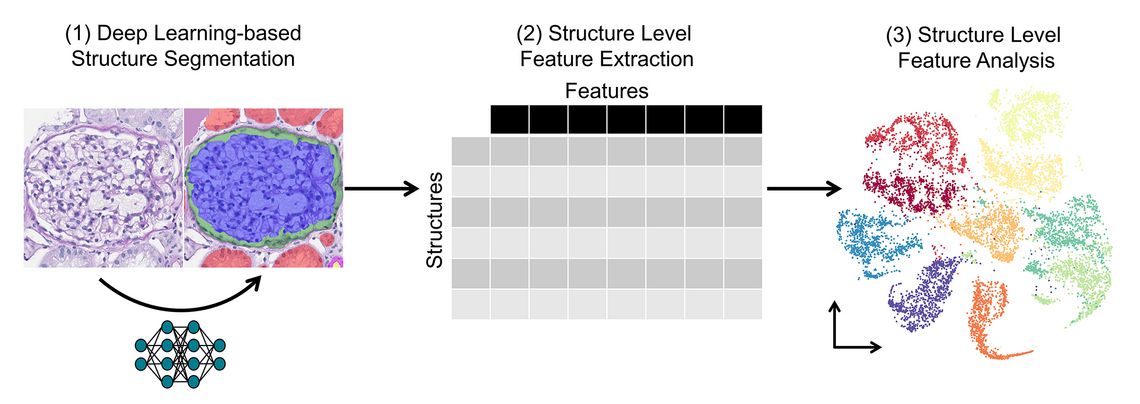
Figure 1: Pathomics analysis workflow overview. (1) Using a structure segmentation model, instances of histological structures are identified in digital histopathology images. (2) A large number of features, i. e., properties are extracted per structure. That way, large datasets often containing feature data of several million histological structures are created. (3) Different techniques are used for downstream analysis of these pathomics datasets, a visualisation of a low-dimensional embedding is given as an example.
The previously mentioned studies focused on larger histological structures, such as glomeruli or tubuli, however, several studies performed cell segmentation as well. Data derived from mononuclear leukocytes and - in addition - larger histological structures were used to predict long- and short-term graft survival in kidney transplants [10]. Mononuclear leukocytes are of high importance in allograft pathology and the prediction model achieved a good performance. Pairs of CD3- and PAS-stained slides were combined using non-linear image registration to allow structure level quantification of CD3-positive cells in kidney transplant pathology, e. g., the CD3-positive cell count per tubule [11]. This important advance allows a much more granular quantification of some Banff-lesions, e. g., tubulitis, than would be feasible using manual scoring. Having to register two whole slide images however slightly reduces large-scale applicability, especially if tissue artefacts are present. This could be circumvented by making structure segmentation models applicable to immunohistochemistry using e. g., generative deep learning models [12].
A score based on segmentation of and feature extraction from glomeruli and podocytes in immunofluorescence images was useful for patient classification and risk stratification in ANCA-vasculitis [13]. Tools to perform similar podocyte identification and quantification both in rodent and human kidney histology were published and made available, enabling use in translational research [14, 15].
These studies show that deep learning-based segmentation and quantification is useful for nephropathology diagnostics and research, and can potentially provide valuable information to clinicians.
Summary & outlook
Significant progress has been made in computational nephropathology, enabling high throughput quantification of histological structures. This may lead to development of new and more granular histological scores used e. g., for risk prediction in the future. A particular advantage of these systems in comparison to end-to-end models is that the extracted data is much better comprehensible, which could strengthen acceptance among pathologists.
The increasing demand for precision in pathology will pose challenges for pathologists, especially considering the increasing workload distributed among an ageing pathologist workforce [16]. It can be expected that automated quantification is more reproducible and faster than manual quantification by a pathologist. Assisting tools such as the systems described in this article thus might be part of the solution for this problem.
Ultimately, prospective studies must evaluate the clinical usefulness of such systems. The first necessary step enabling such trials is digitisation of pathology laboratories. Fully digital pathology centres should cooperate to perform multicentric prospective validation studies of computational pathology tools in the future.